blog
6 Critical Components of Successful Data Governance
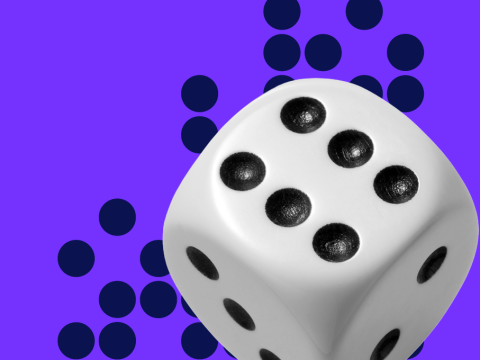
Properly established data governance means that you can make faster and smarter decisions. It sounds simple, but it requires you to build a set of processes and a culture that respects certain key principles.
If you’ve worked with data for any meaningful amount of time, you know that good data depends on good processes – and these don’t tend to exist by chance.
Data and analytics leaders, including Chief Data Officers (CDOs), must implement procedures to ensure their assets are well-governed and aligned with business strategy. Without an established structure, the business will lack the necessary insights to make the best decisions.
But what are the key considerations that you should take into account when building out this kind of structure?
Let’s look at six critical components that form the core pillars of successful data governance.
What is data governance?
Data governance is the process of managing and controlling owned or collected data. It is a set of principles that ensures data quality within a company and also clarifies where ownership and accountability of data assets falls.
In short, it defines who can take what action, upon what data, in what situation, using what method.
For large organizations or companies that are into data management, a documented data governance strategy is the key to ensuring that data is available, usable, and secure.
A company’s data governance documentation will generally cover:
- Policies
- Processes
- Roles
- Responsibilities
- Metrics
These factors form the basis of your data governance framework or program.
Documenting the consistent, common processes and responsibilities means that your data governance strategy is more easily communicated and understood within your business.
Your business drivers will highlight what data needs to be carefully controlled as part of your data governance strategy, as the results shall follow the expected benefits of this effort.
For example, consider those entities that are into medical insurance or health-related organizations or companies. Data privacy is crucial and companies in these industries must comply with regulations such as HIPAA and GDPR.
In this case, the business driver for your data governance strategy ensures privacy. Therefore, patient data will need to be managed securely as it flows through your business. Retention requirements (e.g. history of who accessed or changed what information and when) need to be defined to ensure compliance with relevant government requirements.
6 critical components of successful data governance
Data governance is about standards, policies, and reusability based on its models. But successful data governance rests on six critical components. These are the core pillars of data governance.
Let’s explore each one in more detail.
1. Data architecture
Data architecture is the heart and soul of data governance.
If the architectural design has flaws, this will lead to poor quality or corrupted data. Whatever has been digested and processed, the results cannot be relied on and this affects both the insights analyzed by the company and, in turn, the business goals.
According to The Open Group Architecture Framework (TOGAF), Data Architecture describes the structure of an organization’s logical and physical data assets and data management resources.
Usually the purview of data architects, it is an offshoot of enterprise architecture that unravels the mystery between a series of components covered by data governance.
Those components are the models, policies, rules, and standards that govern the collection, storage, arrangement, integration, and use of data within an organization.
Data architecture explains where data exists and how it travels throughout the organization (either private or egress and ingress data) and its systems. It also highlights any changes and transformations made as data moves from one system to the next.
It will generally use data inventory and data flow diagrams to provide the information and tools the Data Governance Team (DGT) needs to make informed decisions about data policies and standards.
In fact, in many instances business stakeholders say they’d like to better understand the data landscape and how it moves across the organization. DGT’s role in educating the organization on this information and overlaying it with architectural policies and standards helps to ensure data accuracy and integrity throughout its life cycle.
2. Data quality
Your data quality reflects on how it is being collected, planned, analyzed, and processed.
As data governance oversees the quality of the data, the DGT is responsible for identifying when data is corrupt, stale, or inaccurate. Old or stale data can be archived or purged if no longer needed.
High quality data means the organization has access to better, and more accurate, information. In turn, this drives better decisions and better behaviors through the policies that are implemented based on that data.
Policies that are implemented based on high quality data help to maximize the investment the organization has in data and analytics. They can also help to feed the organization’s content decisions, from multimedia, business emails, and so on.
Despite this, 42% of data and analytics leaders do not assess, measure or monitor their data and analytics governance – according to a 2020 Gartner survey. Meanwhile, those who said they do measure governance activity are mainly focused on achieving compliance-oriented goals.
This comes as quite a surprise. It’s clear that strong data governance enables companies to make faster and smarter business decisions, informed by accurate data and analytics.
DGTs need to ensure that high-quality data is available across the entire organization. When done right, this helps to improve the available intelligence that guides key decisions as the business grows.
Data quality is not the only element to be maintained, however. You also need to consider cost.
In simple terms: the bigger the mess, the more expensive the clean up.
This is why it’s important to have the correct rules and processes in place across the entire journey of your data, from the first moment it’s collected.
It may sound obvious, but 58% of organizations say that understanding source data quality is one of the most serious bottlenecks in their data value chain.
It’s also worth noting that the same survey found that automating and matching business terms with data assets, as well as documenting lineage down to the column level, are critical steps to optimizing data quality.
3. Data management
Data management comes down to asking yourself (and answering, of course) two important questions:
- What data do we need to manage?
- Where shall it reside?
For example, based on the type(s) of data you are collecting, does it make sense to store that data on-prem or would it be more valid to have that data reside on a third-party such as public cloud? In some specific scenarios, you might even explore a multi cloud setup or a hybrid environment.
Once you have answered these two high-level questions, you can then build out a full data management action plan.
Your data management plan is essentially how you will execute your data governance strategy.
It sets the specific actions and tasks that need to be carried out in line with the responsibilities, standards, and policies of the data governance strategy. For example, it can cover common tasks such as:
- Creating Role-Based Access rules (RBAC) which sets the level of access for data
- Implementing database rules in alignment to the data governance policy
- Establishing and maintaining data security to comply with what the DGT or CDO has identified for the data owned by the organization
- Taking the appropriate measures to minimize risk associated with storing sensitive data
- Creating a system for master data management, which is a single view of data across the enterprise.
With a data management plan in place, you will have documented methods for accessing, integrating, storing, transferring, and preparing data for analytics.
Remember: effective data governance grows out of data management maturity.
4. Data governance software and tools
You can use various software and tools to help your organization automate and manage the elements of data governance.
In fact, it could be argued that successful data governance cannot be achieved without the use of proper tools to assist in certain areas.
The exact toolset that you land on depends largely on the specifics of your business, the types of data you deal with, and the resources you have available.
But generally speaking, data governance tools can help you in 4 key ways:
- Creation of data catalogs and business glossaries
- Data mapping and classification
- Workflow management and collaboration
- Process documentation and development of policies
When deciding on the software and tools that you will use, keep in mind where your organization needs the most assistance.
The aim is to maintain a consistent set of policies, processes, and owners for your data assets so that you can monitor, manage, and control data movement effectively.
5. Security
Global ransomware damage costs will exceed $265 billion by 2031.
Just let that number sink in a second.
It’s easy to imagine how much impact this kind of security breach can have on a company’s financial health – not to mention the additional effects on brand reputation, consumer trust, and so on.
And ransomware is only one kind of security breach that an organization may be exposed to.
For this reason, security must play a central role in successful data governance. CDOs and/or DGTs must ensure that data security is systematically architectured to provide clarity on:
- Where data comes from
- Where it currently resides
- How it is secured (both at rest and in transit)
- Who has access to it and why
- When and how it’s used
- When and how it is deleted
When you cover these security considerations as part of your organizational data management rules and procedures, it can help prevent potential leaks of sensitive business information or customer data so data doesn’t fall into the wrong hands.
As the amount of data you handle grows, this can become a real challenge.
For example, many legacy platforms and bigger organizations with rich data tend to create siloed data. Over time, it becomes harder to determine where the data came from in the first place. What’s more, the silos are often exported to a database where data gets duplicated and combined with other siloed data – making it even more difficult to audit.
Cloud environments offer a different type of challenge. Given that cloud technology is based on the use of untrusted networks, you should leverage zero trust technologies to advance your security posture.
6. Compliance
Data governance and compliance work hand in hand.
After all, without proper data governance, how can you be confident your organization is adhering to all of the regulations that apply to your business?
There are quite a few these days. Different regulations will apply to your business depending on where you are based geographically, which industry you operate in, and the type of data that you handle.
A few high-profile examples include the European Union’s GDPR (General Data Protection Regulation), while in the US you have the well known PCI DSS (Payment Card Industry Data Security Standard), HIPAA (Health Insurance Portability and Accountability Act), and SOX ACT or Sarbanes–Oxley Act of 2002 (also known as the Public Company Accounting Reform and Investor Protection Act).
These regulations generally require organizations to be able to trace data from source up to its obsolescence. You need to be able to identify who has access to it, and know how and where it is used.
Your data governance framework is how you ensure that clear and compliant control processes are in place.
Without it, sensitive information can leak into the wrong hands or be improperly expunged.
You do not want this to happen. It can lead to financial penalties, lawsuits, and even jail time.
The three critical aspects of building an effective data governance strategy are people, processes, and technology. Platforms such as Snowflake offer features that can set controls on data ownership and access, enabling the implementation of rules and procedures for data governance. These include Dynamic Data Masking and Secure Views.
Conclusion
To sum up, data governance is a collection of processes, roles, and measurable metrics that ensure efficient use of data to enable a certain organization to achieve its goals.
All data governance plans should be carefully and strenuously evaluated to ensure that all six critical components are addressed. You should also assign a senior professional such as a Chief Data Officer or Chief Technology Officer to review your data governance plan periodically.
Stay on top of all things data governance by subscribing to our newsletter below.
Follow us on LinkedIn and Twitter for more great content in the coming weeks. Stay tuned!